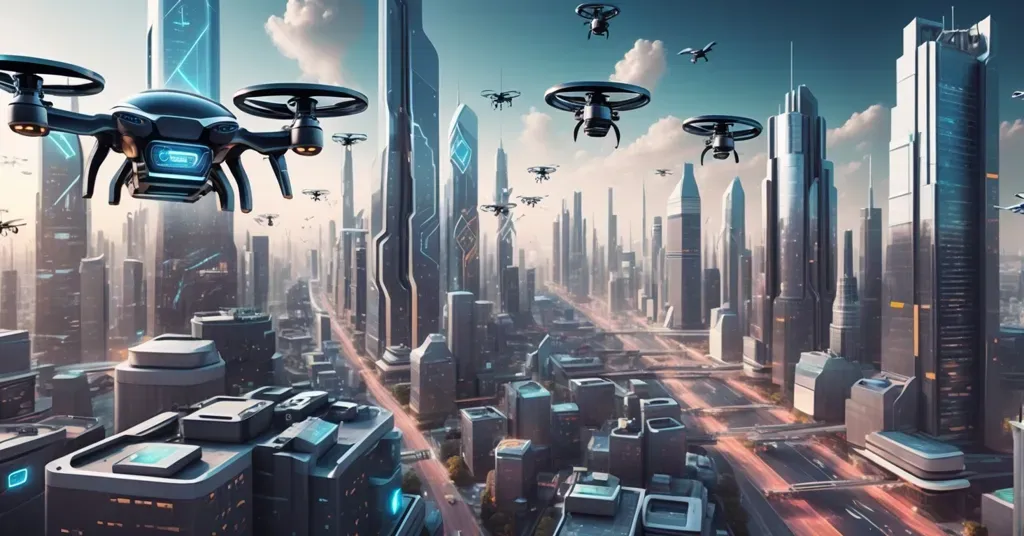
AI and Algorithms: Catalysts for Exponential Growth and Industry Transformation
AI and algorithms are reshaping industries, driving exponential growth, and revolutionizing scientific research. From AlphaFold's protein predictions to strategic business integration, AI's potential is vast but requires careful implementation to overcome biases and challenges.
The transformative potential of artificial intelligence (AI) and algorithms is reshaping industries and driving exponential growth. From streamlining operations to revolutionizing scientific research, AI is not just a tool but a catalyst for innovation. Sundar Pichai, CEO of Alphabet, captures this potential vividly:
"Artificial intelligence could have more profound implications for humanity than electricity or fire."
This bold claim is echoed by other industry leaders, such as Elon Musk, who warns:
"Companies have to race to build AI, or they will be made uncompetitive. Essentially, if your competitor is racing to build AI, they will crush you."
Peter Diamandis, a prominent futurist, further emphasizes the urgency of AI adoption:
"There will be two kinds of companies at the end of this decade: those who are fully utilizing AI, and those who are out of business."
AI's impact is not limited to individual businesses. The World Economic Forum predicts that nearly 25% of jobs will be disrupted by AI in the next five years, signaling a significant shift in the job market. On the economic front, PwC Global estimates that AI could contribute a staggering $15.7 trillion to the global economy by 2030. These projections underline the necessity for organizations to integrate AI into their operations, particularly in large-scale initiatives like Staff on Demand and leveraging crowds and communities for innovation.
Understanding AI and Algorithms
At its core, an algorithm is a set of step-by-step instructions designed to automate tasks or solve specific problems. AI takes this a step further by enabling these algorithms to exhibit "intelligent" behavior, learning and solving new problems independently. This capability to mimic and even surpass human thinking is what makes AI so powerful. Imagine AI as a genius intern, constantly learning and improving, ready to tackle any challenge you throw its way. For a deeper understanding, explore What are AI and Algorithms?
The Power of AI in Scientific Research: The AlphaFold Case Study
A compelling example of AI's potential is AlphaFold, developed by DeepMind. AlphaFold uses AI to predict protein structures, a crucial challenge in biology. Its success in the CASP competition, where AlphaFold 1 ranked first in 2018 and AlphaFold 2 achieved remarkable accuracy in 2020, showcases the power of deep learning in advancing scientific research. Researchers have described AlphaFold 2's results as:
"astounding" and "transformational."
By training on over 170,000 proteins, AlphaFold leverages an attention network, a form of deep learning, to make precise predictions. This opens new avenues for understanding biological processes and developing treatments. To see more on this, read about the Case Study - AlphaFold - The Power of AI and Algorithms in Predicting Protein Structures. For the latest developments in this field, check out recent developments in AI for protein structure prediction post-AlphaFold 2.
Key Concepts in AI and Algorithms
To fully grasp the landscape of AI and algorithms, it's essential to understand key concepts. Consider these terms:
- Narrow AI: AI that can solve only the tasks it is trained for.
- Artificial General Intelligence (AGI): AI capable of understanding any intellectual task a human can do.
- Machine Learning: Enables machines to deduce concepts from data without explicit programming.
- Neural Nets: Machine-learning models based on human brain neurons.
- Deep Learning: Uses multiple processing layers to extract features from data.
- Generative Adversarial Networks (GANs): Involves a generator and discriminator working adversarially.
- Natural Language Processing (NLP): Focuses on human language interaction with computers.
- Federated Learning: Decentralized machine learning that preserves data privacy.
- Large Language Models (LLMs): Advanced models for natural language tasks, trained on massive datasets.
These technologies are advancing AI's capabilities, enabling it to tackle increasingly complex problems. For a comprehensive overview, refer to Description of AI and Algorithms - Key Concepts.
Overcoming Human Cognitive Biases with AI
AI promises to revolutionize decision-making by overcoming human cognitive biases such as availability bias, the bandwagon effect, and hindsight bias. However, AI systems can also inherit biases from their programming and have their own set of potential flaws. This highlights the importance of careful implementation and monitoring to ensure AI's benefits are fully realized without unintended consequences. Learn more about Why AI? Overcoming Human Cognitive Biases.
Strategic AI Integration for Businesses
To harness AI's potential, businesses must develop strategic approaches to integration. Here are some practical steps:
- Identify areas where AI can add the most value.
- Invest in talent and technology to support AI initiatives.
- Foster a culture that embraces continuous learning and adaptation.
Consider how companies like Amazon and Netflix use AI for personalization, transforming customer experiences and driving business growth. For insights into strategic integration, visit Strategic AI Integration for Businesses.
Key Takeaways and Questions
Here are some key insights and questions to consider:
- What strategies can companies implement to integrate AI effectively?
Companies can start by identifying areas where AI can add the most value, investing in talent and technology, and fostering a culture that embraces continuous learning and adaptation.
- How can businesses prepare for the potential job disruptions caused by AI?
Businesses should focus on reskilling and upskilling their workforce, promoting a culture of lifelong learning, and integrating AI in ways that complement rather than replace human roles. For more on this topic, see Impact of AI on Job Market.
- What are the ethical considerations companies should address when adopting AI technologies?
Companies must prioritize transparency, fairness, and accountability in AI systems, ensuring they address privacy concerns, mitigate biases, and establish clear ethical guidelines for AI use.
- How do algorithms contribute to the functionality of AI systems?
Algorithms form the backbone of AI systems, providing the structured processes that enable AI to perform tasks, learn from data, and make decisions. For more on this, visit Artificial Intelligence and Algorithms.
- What distinguishes AI from traditional software programming?
AI can learn and adapt to new situations without explicit programming, whereas traditional software follows predetermined instructions without the ability to adapt independently.
- How might the advancements in protein structure prediction impact drug development?
By accurately predicting protein structures, AI can accelerate drug discovery by identifying potential drug targets more efficiently and reducing the time and cost of development. Explore AI in Drug Development for further insights.
- What are the potential future applications of AI in other scientific fields?
AI could revolutionize fields like climate science, materials science, and genetics by analyzing complex data sets, predicting outcomes, and optimizing processes.
- How do different AI models like Narrow AI and AGI impact their potential applications?
Narrow AI is suited for specific, well-defined tasks, whereas AGI could theoretically handle a broad range of tasks, offering versatile applications across various domains.
- What are the privacy implications of using federated learning in AI development?
Federated learning can enhance privacy by keeping data on local devices and only sharing model updates, but it requires robust security measures to prevent data leakage and ensure model integrity.
- How can organizations ensure that AI systems are free from inheriting human biases?
Organizations must use diverse and representative training data, implement bias detection and mitigation techniques, and regularly audit AI systems for fairness and accuracy.
- What steps can be taken to address the potential flaws in AI systems?
Continuous monitoring, rigorous testing, and the integration of human oversight can help identify and correct flaws in AI systems, ensuring they operate reliably and ethically.
Final Thoughts
The urgency to embrace AI is clear, and the potential for exponential growth and innovation is immense. As you navigate the transformative landscape of AI and algorithms, consider these insights and take proactive steps to harness their power within your organization. Explore the concepts of Exponential Organizations further to unlock your company's full potential in this era of technological advancement. For more on the broader implications of AI, see Artificial Intelligence and Exponential Growth.
- AI can drive exponential growth and revolutionize industries.
- The economic impact of AI is projected to be significant.
- Understanding AI concepts is crucial for leveraging its potential.
- Careful implementation is necessary to mitigate AI's challenges and biases.
Take the first step towards exponential growth by integrating AI into your strategic planning. Explore our resources on Exponential Organizations to learn more and unlock your organization's full potential. For community discussions on algorithms in research, visit Algorithms and Scientific Research.
ExO Insight Newsletter
Join the newsletter to receive the latest updates in your inbox.